Reimagine SAP Process Mining with AI Agents
Introduction
Process Mining is a technique that leverages data from IT systems to analyze, monitor, and improve business processes. In an era where data-driven decision-making is paramount, Process Mining offers a unique lens through which organizations can understand their operations' reality.
Today, with the rise of Agentic AI systems — intelligent entities that can autonomously analyze, decide, and act — and Generative AI technologies that contextualize patterns and generate insights beyond traditional analytics, Process Mining is entering a transformative era. This blog post delves into the intricacies of Process Mining, its applications, benefits, and how it can transform business operations through the integration of autonomous intelligence and generative capabilities.
What is Process Mining?
Process Mining is the analytical approach that extracts knowledge from event logs produced by IT systems. These logs capture the digital footprint of business processes, allowing organizations to reconstruct and analyze the actual flow of operations. Unlike traditional process analysis methods that rely on assumptions and interviews, Process Mining provides a factual representation of how processes are executed.
Now, with GenAI-powered cognitive models embedded into modern process mining tools, insights are no longer limited to what’s visible in the logs. These systems can generate explanations, simulate optimizations, and even suggest new process variants based on best practices across industries.
Key Components of Process Mining
- Event Logs: The foundation of Process Mining lies in event logs. These logs record every action within a system, such as the creation of a sales order, approval of a purchase requisition, or delivery of a product. Each log entry typically includes a timestamp, the event type, and the user or system that performed the action.
- Process Discovery: This involves using event logs to reconstruct the end-to-end process flow. The result is a visual representation of the "As-Is" process, showing how activities are sequenced and interconnected.
- Conformance Checking: This step compares the actual process execution against predefined models or standards. It helps identify deviations, compliance issues, and areas where the process does not align with intended designs.
- Process Enhancement: By analyzing the reconstructed process, organizations can identify bottlenecks, inefficiencies, and opportunities for improvement. This leads to data-driven process enhancement initiatives.
Modern platforms like KTern.AI use Agentic AI agents to monitor these process components continuously, making autonomous decisions to flag anomalies, initiate root-cause diagnostics, or trigger automation workflows without manual intervention.
How Process Mining Works
Step-by-Step Process
- Data Extraction: The first step is to extract event logs from various IT systems. These systems can include ERP platforms like SAP, CRM systems like Salesforce, or service management tools like ServiceNow.
- Data Preprocessing: The extracted data is cleaned and preprocessed to ensure it is in a suitable format for analysis. This step involves handling missing values, removing duplicates, and standardizing data formats.
- Process Discovery: Using specialized algorithms, the event logs are analyzed to discover the underlying process flow. This step generates a visual model of the process, highlighting variants, bottlenecks, and rework loops.
- Analysis and Diagnostics: The discovered process model is analyzed to identify inefficiencies, compliance issues, and areas for improvement. This step involves calculating key performance indicators (KPIs) and generating insights.
With Generative AI, this goes one step further — enabling automated creation of narrative reports, root-cause hypotheses, and predictive what-if scenarios. - Process Improvement: Based on the analysis, organizations can implement changes to optimize their processes. This may involve automating repetitive tasks, redesigning workflows, or introducing new technologies.
- Continuous Monitoring: Process Mining is not a one-time activity. Organizations can set up agentic monitoring loops to track process performance over time and ensure sustained improvements, while dynamically adapting to evolving conditions.
Applications of Process Mining
- Procure-to-Pay Process
The Procure-to-Pay (P2P) process is a critical business function that involves purchasing goods and services. Process Mining can help organizations analyze the P2P process to identify delays, inefficiencies, and compliance issues. For example, it can highlight vendors that cause longer wait times or invoices that are blocked due to missing Goods Receipt Notes (GRNs).
Agentic systems can now autonomously recommend vendor switches, escalate delays, and suggest policy changes in procurement cycles.
- Order-to-Cash Process
The Order-to-Cash (O2C) process involves fulfilling customer orders and collecting payments. Process Mining can reconstruct the O2C process to identify bottlenecks, such as delays in order processing or payment collection. It can also highlight variants in the process, such as different payment methods or order fulfillment channels.
GenAI tools like KTern.AI can enrich this analysis by generating customer personas, predicting payment behavior, and even recommending personalized workflows based on customer segments.
Benefits of Process Mining
- Improved Process Visibility: Process Mining provides a comprehensive view of business processes, enabling organizations to understand the actual flow of operations. This visibility is crucial for identifying inefficiencies, bottlenecks, and areas for improvement.
- Enhanced Compliance and Audit Readiness: By comparing actual process execution against predefined standards, Process Mining helps organizations ensure compliance with regulatory requirements and internal policies. It provides a digital audit trail that can be used for audit preparation and continuous monitoring.
- Cost Reduction and Efficiency: Process Mining helps organizations identify and eliminate unnecessary steps, automate repetitive tasks, and improve process accuracy. This leads to reduced costs, improved efficiency, and enhanced customer satisfaction.
- Data-Driven Decision Making: Process Mining provides factual insights based on actual data, enabling organizations to make data-driven decisions.
With GenAI copilots, these decisions are now supplemented by intelligent recommendations, contextual narratives, and suggested next actions, amplifying human decision-making.
SAP Process Mining
What is SAP Process Mining?
SAP Process Mining is the application of process mining techniques specifically to SAP systems. It involves extracting event logs from SAP modules, such as FI (Financial Accounting), MM (Materials Management), and SD (Sales and Distribution), to analyze the actual execution of business processes.
What is KTern.AI Process Mining?
KTern.AI’s Process Mining is an X-ray view into your SAP processes — not how they were designed to run, but how they actually run. This real-world visibility is critical for digital transformation leaders, SAP PMOs, and operational teams driving clean core initiatives.
Process Explorer: From Start to Finish
KTern.AI automatically mines your SAP transactional logs to reconstruct the real O2C flow. In the visualization:
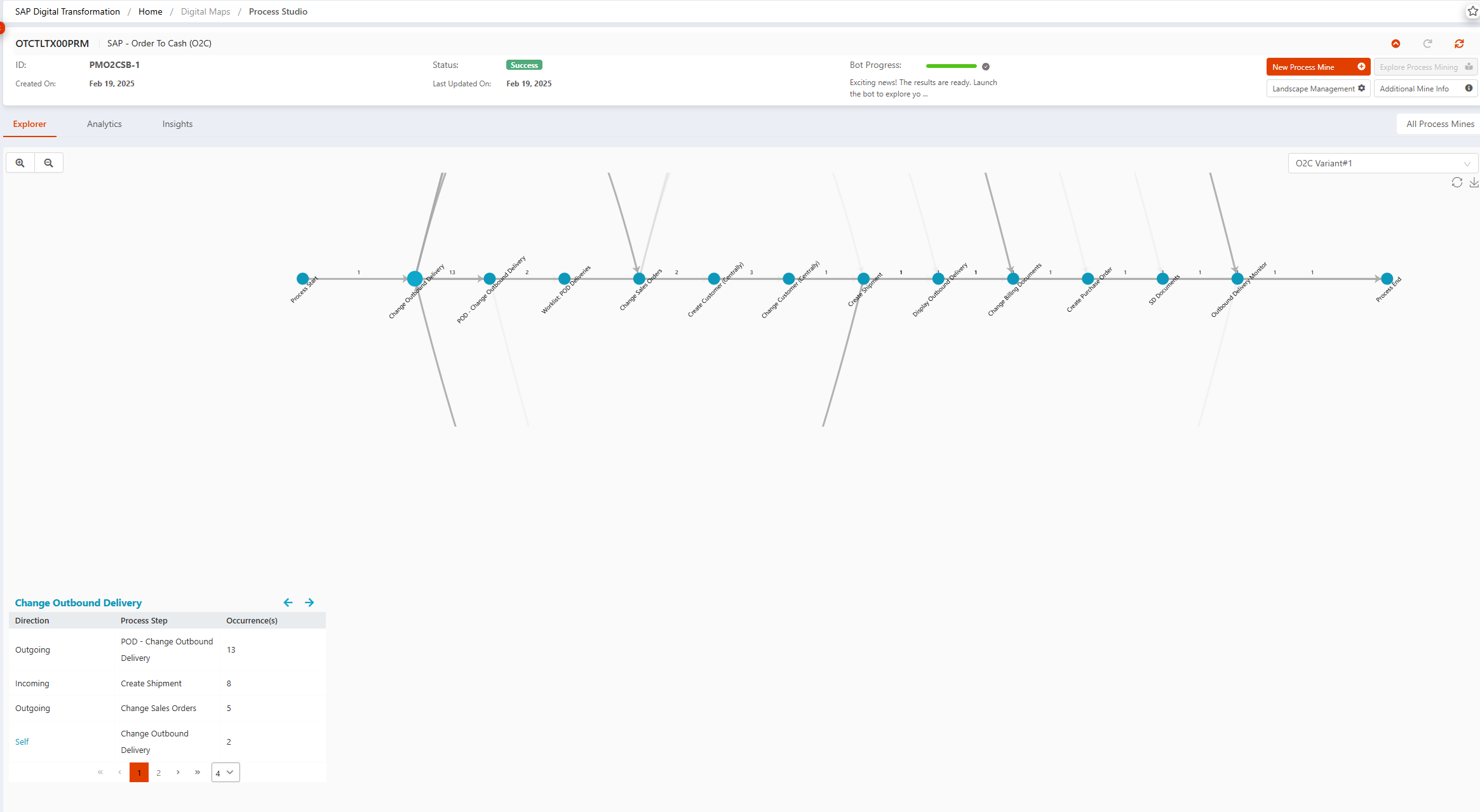
- Each node is a business event (e.g., Sales Order Creation, Delivery Processing, Billing).
- Each arrow represents the actual sequence in which these events occurred.
With GenAI enhancements, the explorer not only maps the process but also generates explanations for anomalies, summaries for executives, and intelligent playbooks for optimization.
KTern.AI Process Analytics: A Deep Dive
Each of the following analytics capabilities becomes significantly more powerful when backed by Generative AI summarization and Agentic task orchestration, which ensure proactive alerts, automated follow-ups, and human-in-the-loop actions:
1. Business Process Steps
Insight: KTern.AI provides a detailed breakdown of each step involved in a business process.
Why It's Important: Understanding the sequence and nature of each process step is foundational to identifying areas for improvement. It helps in visualizing the end-to-end process flow and ensures that all stakeholders have a clear understanding of how work is executed.
Value Added: This clarity enables teams to align on process standards, identify unnecessary steps, and streamline operations for better efficiency.
2. Average End-to-End (E2E) Process Duration
Insight: This metric calculates the average time taken for a process to complete from start to finish.
Why It's Important: Knowing the E2E duration helps in setting benchmarks and identifying processes that take longer than expected. It is a critical KPI for measuring process performance.
Value Added: By tracking this metric, organizations can set targets for process improvement initiatives and measure the impact of changes over time.
3. Top Business User
Insight: Identifies the users who are most actively involved in the business process.
Why It's Important: Understanding who the key users are can help in optimizing workload distribution and identifying potential bottlenecks caused by user-specific issues.
Value Added: This insight aids in better resource allocation, training, and support for high-activity users, ensuring smoother process execution.
4. Top Process Step
Insight: Highlights the most frequently executed step in the process.
Why It's Important: Frequently executed steps are often critical to the process and can be targets for automation or optimization.
Value Added: By focusing on these steps, organizations can achieve significant efficiency gains and reduce manual effort.
5. Process Step with the Most Rework
Insight: Identifies the process step that requires the most revisions or repetitions.
Why It's Important: High rework indicates inefficiencies, errors, or lack of standardization. Addressing these steps can lead to substantial improvements in process quality and speed.
Value Added: Reducing rework lowers operational costs, improves accuracy, and enhances overall process reliability.

6. Activity Frequency
Insight: Shows the number of times each business process activity is performed.
Why It's Important: Activity frequency helps in understanding the workload and identifying high-volume tasks that may benefit from automation or process redesign.
Value Added: This insight enables data-driven decisions on where to focus improvement efforts for maximum impact.
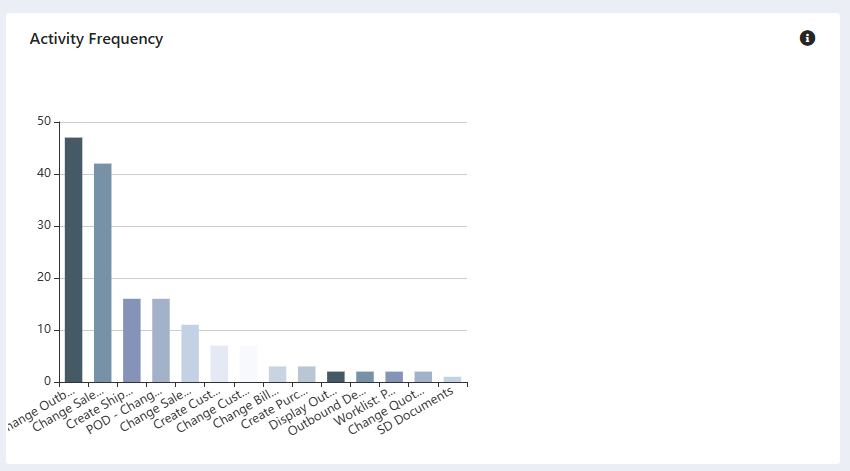
7. User Frequency
Insight: Indicates the count of process steps each user is involved in.
Why It's Important: Monitoring user frequency helps in balancing workloads and ensuring that no single user becomes a bottleneck.
Value Added: This data aids in optimizing resource allocation, reducing burnout, and improving overall team productivity.
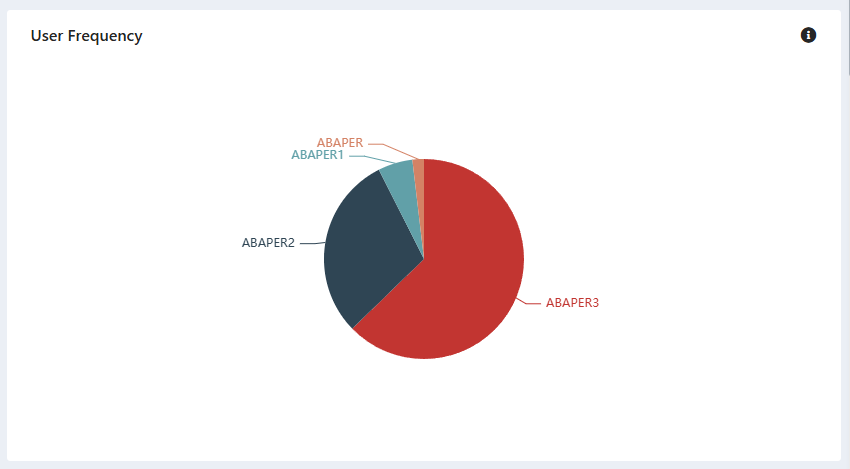
8. Throughput Time vs. Total Waiting Time
Insight: Compares the average time tasks take to progress (throughput time) with the total delays experienced at each step (waiting time).
Why It's Important: High waiting times can indicate inefficiencies, resource constraints, or approval delays. Understanding these metrics helps in identifying and addressing bottlenecks.
Value Added: By reducing waiting times, organizations can improve process flow, decrease cycle times, and enhance customer satisfaction.
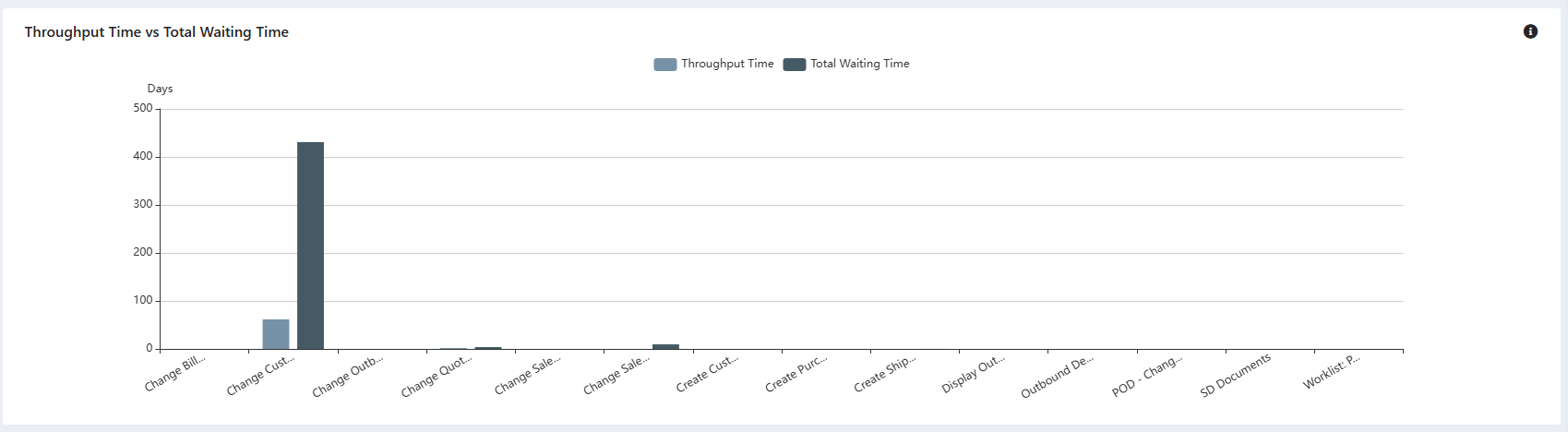
9. Process Step Rework Count
Insight: Counts the total number of times each process step is repeated.
Why It's Important: High rework counts signal potential issues such as incomplete data, errors, or lack of clarity in process instructions.
Value Added: Addressing steps with high rework counts can lead to significant improvements in process efficiency and quality.
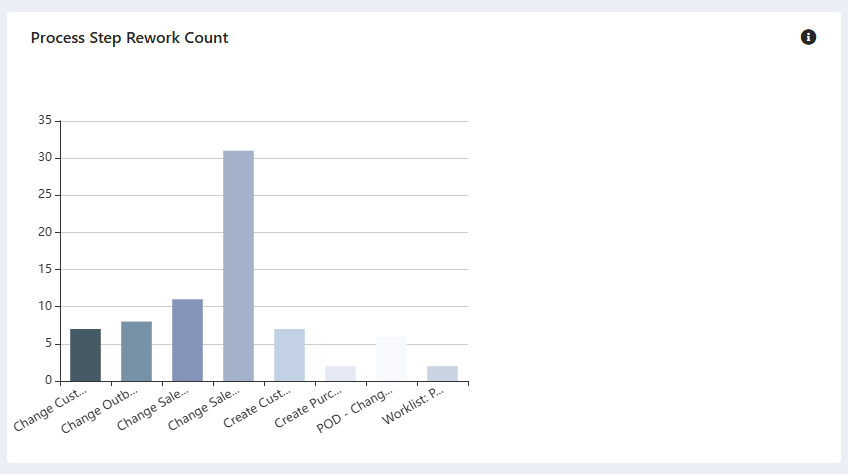
10. User Rework Count
Insight: Shows the cumulative count of rework instances by each user.
Why It's Important: Identifying users with high rework counts can reveal training needs, workload issues, or process gaps.
Value Added: This insight helps in targeting specific users for support or training, improving overall process execution and reducing errors.
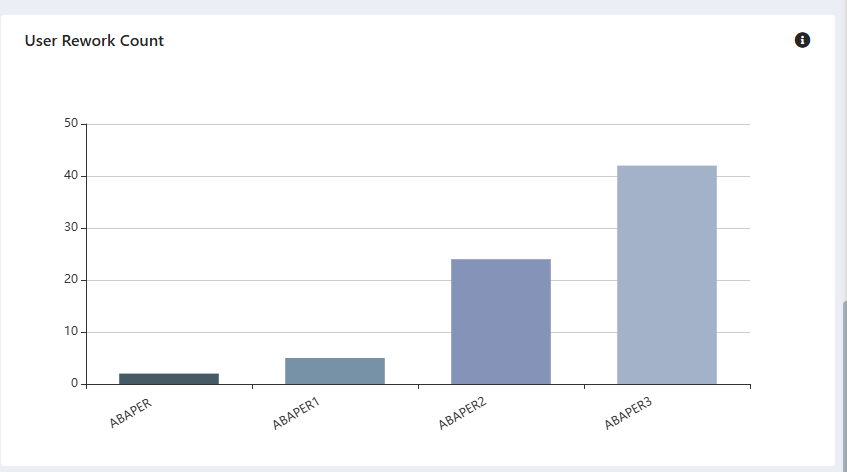
11. Top 20 Cases with Longest Duration
Insight: Lists the top 20 process instances that took the longest to complete.
Why It's Important: Analyzing these cases can reveal patterns or issues that contribute to delays, such as complex transactions or exceptional scenarios.
Value Added: By understanding the root causes of delays in these cases, organizations can implement targeted improvements to reduce process duration and enhance efficiency.
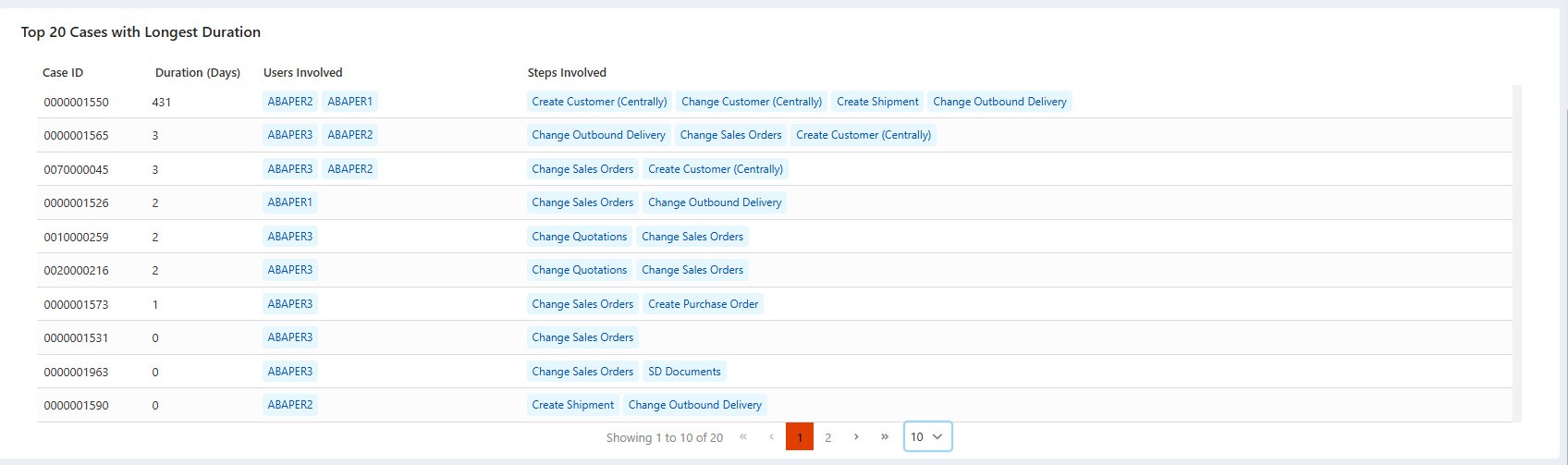
Harnessing AI-Driven Process Insights
1. AI-Driven Process Observations
KTern.AI employs AI to provide real-time observations about business processes, highlighting areas that need attention, along with intelligent recommendations for improvement.
Components:
- Current Observation: AI algorithms analyze event logs to identify patterns, anomalies, and inefficiencies in the current process flow. This includes detecting bottlenecks, compliance gaps, and deviations from standard procedures.
- Recommendation: AI-generated suggestions are tailored to address the observed issues, offering actionable steps to optimize processes and enhance performance.
Why It's Important: AI-driven process observations offer a dynamic and proactive approach to process management. By continuously monitoring and analyzing processes, organizations can quickly identify and rectify issues before they escalate.
Value Added: These insights enable organizations to stay ahead of potential problems, ensuring smooth and efficient process execution. The AI-powered recommendations provide a roadmap for immediate improvements, leading to enhanced operational efficiency and compliance.
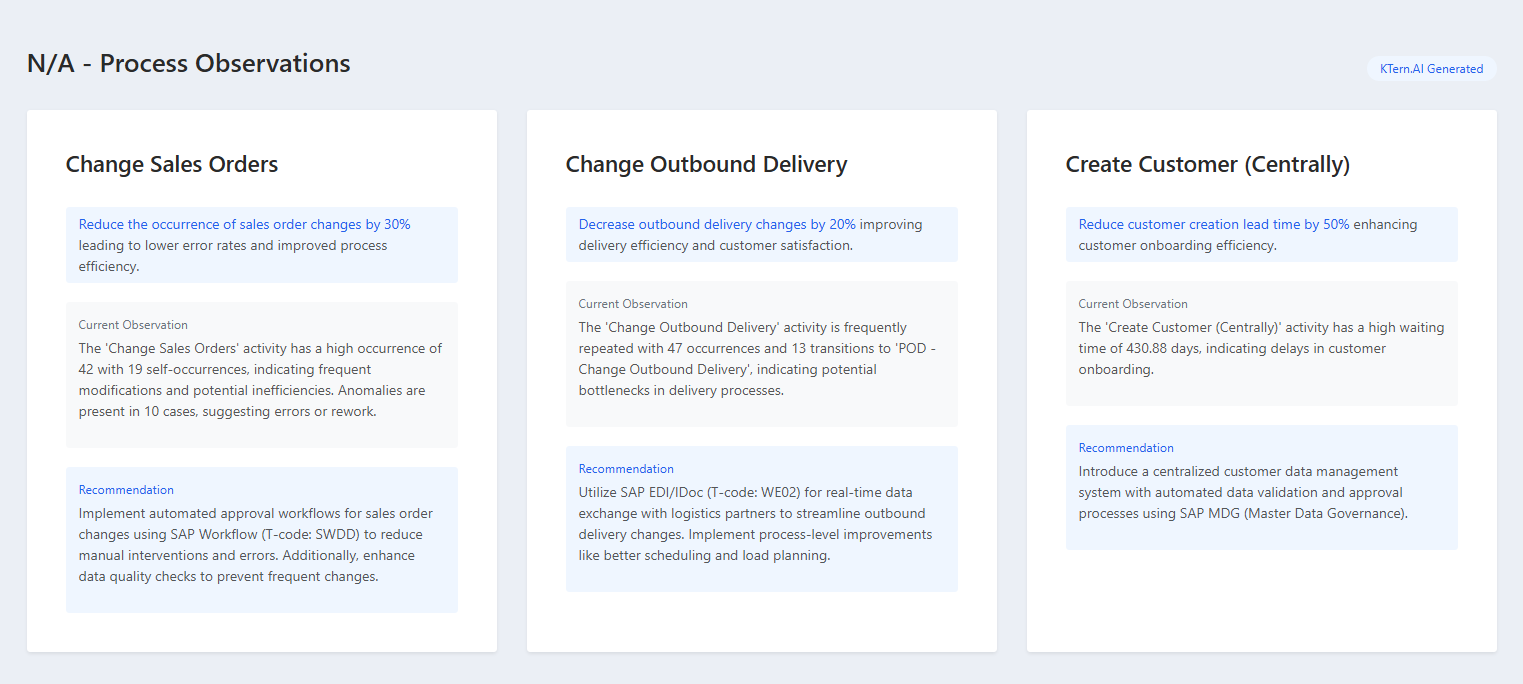
2. AI-Enhanced Key Performance Indicators (KPIs)
KTern.AI leverages AI to track and analyze key performance indicators, comparing current values against benchmarks and providing data-driven recommendations for improvement.
Components:
- Current Value vs. Benchmark: AI algorithms compare current KPI values against industry or internal benchmarks, highlighting areas where the process is underperforming. This involves analyzing historical data and predicting future trends.
- Recommendation: AI-generated suggestions are based on deep learning models that identify the most impactful changes to improve KPIs. These recommendations help organizations achieve their performance targets more effectively.
Why It's Important: KPIs are essential for measuring the effectiveness and efficiency of business processes. AI-enhanced KPI tracking provides a more accurate and predictive analysis, enabling organizations to set realistic targets and drive continuous improvement.
Value Added: By leveraging AI, organizations can gain deeper insights into their KPIs, making informed decisions that drive significant improvements in process performance and overall business outcomes.
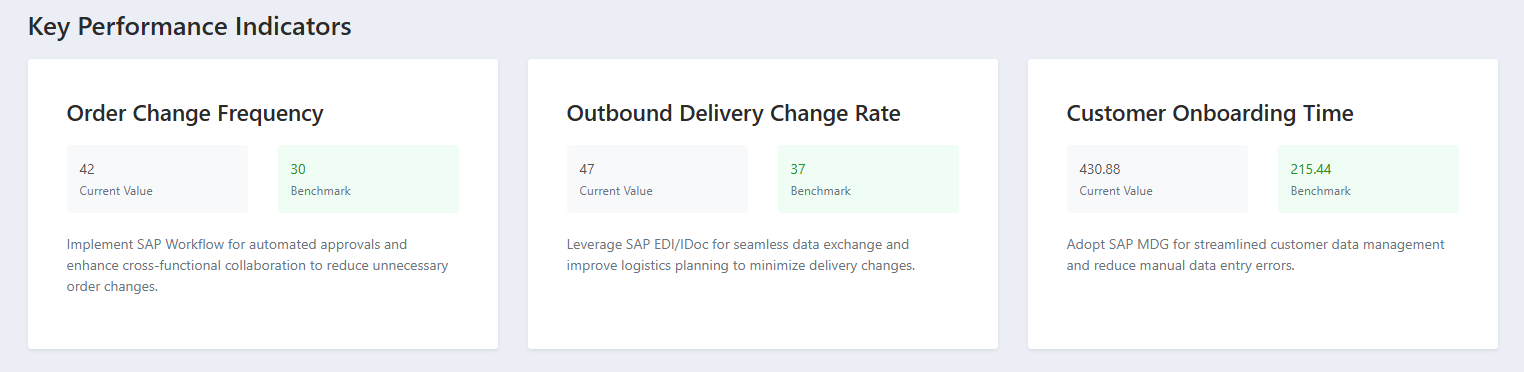
3. AI-Powered Strategic Recommendations
KTern.AI offers AI-powered strategic recommendations based on comprehensive process insights, providing a roadmap for long-term improvements.
Components:
- Description: AI algorithms generate detailed explanations of recommended actions, outlining the steps required to implement changes. These descriptions are based on a thorough analysis of process data and industry best practices.
- Quantifiable Expected Impact: AI models estimate the potential benefits of implementing the recommendations, providing quantifiable metrics such as cost savings, efficiency gains, or improved customer satisfaction. These predictions are based on historical data and machine learning algorithms.
Why It's Important: AI-powered strategic recommendations provide a data-driven approach to process improvement. They help organizations align their process enhancements with broader strategic goals, ensuring that changes deliver measurable results.
Value Added: By implementing these recommendations, organizations can achieve significant and sustainable improvements in process performance. The AI-driven insights enable organizations to make informed decisions that enhance operational efficiency, reduce costs, and drive competitive advantage.
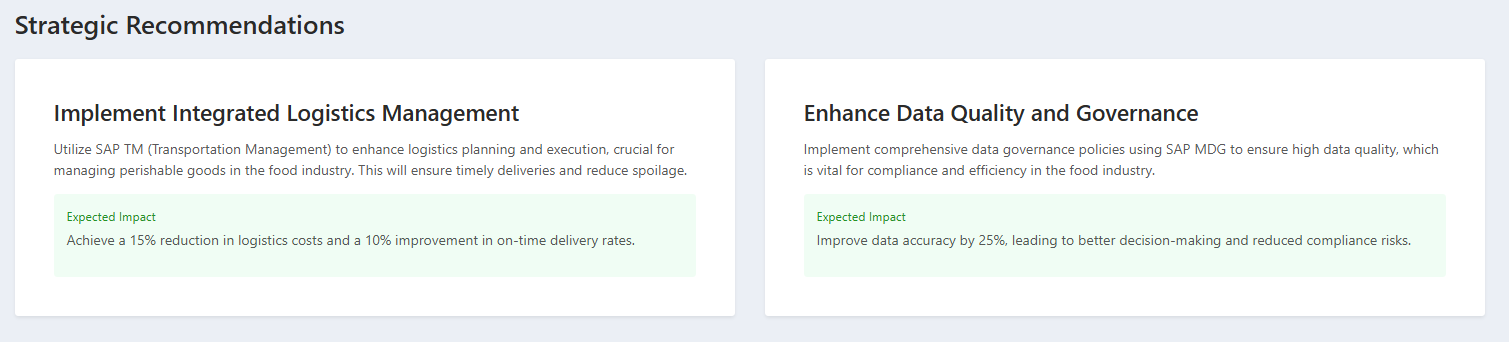
Conclusion
Process Mining has emerged as a transformative technique, enabling organizations to achieve unprecedented levels of efficiency and insight. With the integration of Agentic AI and Generative AI, Process Mining is no longer just about understanding the past; it's about shaping the future.
KTern.AI stands at the forefront of this revolution, embodying the modern AI-first approach to process optimization. By leveraging Agentic AI, KTern.AI can autonomously monitor processes, identify anomalies, and initiate corrective actions without human intervention. This capability ensures that processes are not only optimized but also adaptive to changing conditions, maintaining peak performance in real-time.
Generative AI further amplifies KTern.AI's capabilities by providing contextual insights, creating innovative solutions, and simulating optimizations that go beyond traditional analytics. This enables organizations to explore new process designs, predict future trends, and make data-driven decisions that drive continuous improvement.